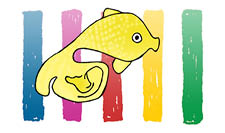
TAUS has kindly requested a blog series on current topics in automatic translation. As an opening salvo, here’s a sneak preview of the forthcoming TAUS report on speech-to-speech translation (S2ST). The report, co-authored by Alex Waibel, Andrew Joscelyne, and myself, will attempt a broad view of the field’s past, present, and future. For this appetizer, though, we’ll restrict our view to a brief snapshot – a selfie, if you like – of selected technical accomplishment at the current state of the art. (The report will include interviews with several additional participants.)
- Google Translate mobile app:
- Speed: Barring network delays, speech recognition and translation proceed and visibly update while you speak: no need to wait till you finish. When you do indicate completion by pausing long enough – about a half second – the pronunciation of the translation begins instantly.
- Automatic language recognition: Manually switching languages is unnecessary: the application recognizes the language spoken – even by a single speaker – and automatically begins the appropriate speech recognition and translation cycle. End-of-speech recognition, too, is automatic, as just explained. As a result, once the mic is manually switched on in automatic-switching mode, the conversation can proceed back and forth hands-free until manual switch-off. (Problems will arise if speakers overlap, however.)
- Noise cancellation: Speech recognition on an iPhone works well in quite noisy environments – inside a busy store, for instance.
- Offline capability: Since speakers, and especially travelers, will often need speech translation when disconnected from the Internet, Google has introduced an offline version to download a given language pair onto a smartphone for offline use. (Jibbigo – see below – had previously introduced exclusively offline apps.)
- Dynamic optical character recognition: This capability isn't exactly speech translation, but it is now a well-engineered-and-integrated part of Google’s translation suite. The app can recognize and translate signs and other written material, with the translation text replacing the source text within the image scene as viewed through the smartphone’s camera viewer. The technology extends considerable previous research in optical character recognition (OCR), and particularly work by WordLens, a startup acquired by Google in 2014 that had performed the replacement trick for individual words. The current version handles entire segments, and dynamically maintains the positioning of the translation when the camera and source text move.
- Skype Translator (powered by Microsoft):
- Telepresence: Microsoft and its Skype subsidiary weren't the first to offer speech translation in the context of video chat: as one example, by the time Skype Translator launched, Hewlett-Packard had for more than two years already been offering a solution in its bundled MyRoom application, powered by systems integrator SpeechTrans, Inc. And speech translation over phone networks – but lacking video or chat elements – had been inaugurated experimentally through the C-STAR consortium and commercially through two Japanese efforts (NEC's mobile device for Japanese-English in 2006 and the Shabete Honyaku service from ATR-Trek in 2007). But the launch of Skype Translator had great significance because of its larger user base and consequent visibility – it exploits the world’s largest telephone network – and in view of several interface refinements.
- Spontaneous speech: The Microsoft translation API contains a dedicated component, TrueText, to “clean up” elements of spontaneous speech – hesitation syllables, errors, repetitions – long recognized as problematic when delivered to an SLT system’s translation engine. The component’s goal, following a long research tradition, is to translate not what you said, stutters and all, but what you meant to say.
- Overlapping voice: Borrowing from news broadcasts, the system begins pronunciation of its translation while the original speech is still in progress. The volume of the original is lowered so as to background it. The aim of this “ducking” is to encourage more fluid turn-taking. The hope is to make the technology disappear, so that the conversation feels maximally normal to the participants.
- Interpreting Services InterACT (Waibel, KIT/CMU):
- Simultaneous Interpreting Services: Following the release of Jibbigo as the first network-free mobile SLT app, the team at InterACT (the International Center for Advanced Communication Technologies, Waibel et al.) pioneered simultaneous interpreting of lectures. The first lecture translator was demonstrated in 2005 [Fügen et al., 2006, 2007]. The technology was deployed in 2012 as a Lecture Interpretation Service that now operates in several lecture halls at Karlsruhe Institute of Technology (KIT). Target users are foreign students and the hearing-impaired.
- Continuous online interpretation streaming: During a lecture, speech is streamed over WiFi to KIT servers that process subscribed lectures. Speech recognition and Translation is performed in real time, and output is displayed via standard Web pages accessible to students.
- Off-line browsing: Transcripts are offered offline for students’ use after class. Students can search, browse, or play segments of interest along with the transcript, its translation, and associated slides.
- Speed: The Lecture Translator operates at very low latency (time lag). Transcriptions of the lecturer’s speech are displayed instantaneously on students’ devices as subtitles, and translations appear incrementally with a delay of only a few words, often before the speaker finishes a sentence.
- Readability: To turn a continuous lecture into readable text, the system removes disfluencies (stutters, false-starts, hesitations, laughter, etc. – compare Microsoft’s TrueType, above), and automatically inserts punctuation, capitalization, and paragraphs. (Speakers needn’t pronounce commands like “Comma,” “Cap That,” or “New Paragraph.”) Spoken formulas are transformed into text where appropriate (“Ef of Ex” → f(x)). Special terms are added to ASR and MT dictionaries from background material and slides.
- Multimodality: Beta versions include translation of slides; insertion of Web links to study materials; emoticons; and crowd-editing. They also support alternative outputs: speech synthesis, targeted audio speakers instead of headphones, or goggles with heads-up displays.
- European Parliament: Variants and subcomponents are being tested at the European Parliament to support human interpreters. (A Web-based app automatically generates terminology lists and translations on demand.) The system tracks numbers and names – difficult for humans to remember while interpreting. An “interpreter’s cruise control” has been successfully tested for handling repetitive (and boring) session segments like voting.
Hope these hors d'oeuvres have whetted your appetite! The TAUS S2ST Report is expected in March, 2017; and future blog posts will range far and wide.
References
Fügen, Christian, Muntsin Kolss, and Alex Waibel. 2006. “Open Domain Speech Translation: From Seminars and Speeches to Lectures.” In Proceedings of the TC-Star Workshop on Speech-to-Speech Translation, TC-STAR-WS 2006. Barcelona, Spain, June 19, 2006.
Fügen, Christian, Alex Waibel, and Muntsin Kolss. 2007. “Simultaneous Translation of Lectures and Speeches.” Machine Translation (2007) 21: 209.